Machine Learning Feature Store for Fraud and Compliance
Data and ML have become the lifeblood of fraud and AML teams.
Data science is the key tool used in the front line of risk management. Innovative CTOs and their in-house data science teams are realizing unprecedented ROI by customizing ML models to their unique risk profiles.
Many data and ML features lie beyond an organization's boundaries, untapped and underutilized.
3rd parties often return scores but never how they got to that score.
The fraud and compliance squad would love to know how they got to the score and access the underlying data. They might have other ML models they want to pull it into or data they want to combine to assess risk.
What if 3rd party data and ML features worked just like internal tools?
The solution is a feature store. Allowing your data scientists to centralize, simplify, and make accessible machine-learned features from 3rd parties.
For more about how that works let’s discuss
- An example of Machine learned features
- How we generate those features
- How the feature store works
1. Give me an example of Sardine Machine Learned features
At Sardine, we’ve worked hard to create the Sardine ML Featurestore. We give clients direct access to our data warehouse and over 4,000 machine-learned features. But what is a machine-learned feature?
For us, they can be seemingly simple data points like 'User.Country'. The reality is understanding the true country of a user can be complex (if they’re using proxies or a VPN, for example). A client using the Sardine 'User.Country feature is pulling the Sardine insight on that user.
A feature could also include more complex aggregations. For instance, we can determine the count of customers from a given IP address within a day (e.g., CustomerSession.IPV4Aggregations.CustomerAggregation.CustomerCount_1DAY). This might be useful if one particular IP address is creating lots of accounts suddenly.
We also explore network-specific features, like determining if a particular email was previously associated with a fraudulent chargeback within Sardine's network (email.isFraudulent).
Each of these features can be valuable in a myriad of ways. If you understand how they’re generated, you understand how to use them as a data science team.
2. Context: How we Generate Sardine’s ML Features
The Sardine SDK captures device and behavior signals directly from the user's equipment. Picking up information such as device ID, the operating system in use, IP details, and unique user behavior patterns like how you swipe, type, tap, or hold your phone. This powerful set of signals forms a base for machine-learned models and can be accessed via the 1000s of features.
Because these models are pre-trained on Sardine’s data set, we can flag signals like rooted device detection, remote software, anomalies in the OS, and more. The model outputs provide features that act as indicators, insights, and red flags for the fraud and compliance squad.
3. How the Feature Store works.
Feature stores should be an extension of your tech estate.
Nobody wants to walk into a store where they can’t buy anything.
We provide access to data warehouses (Snowflake or Big Query) for data training and through a real-time API for inference. It looks like the picture below.
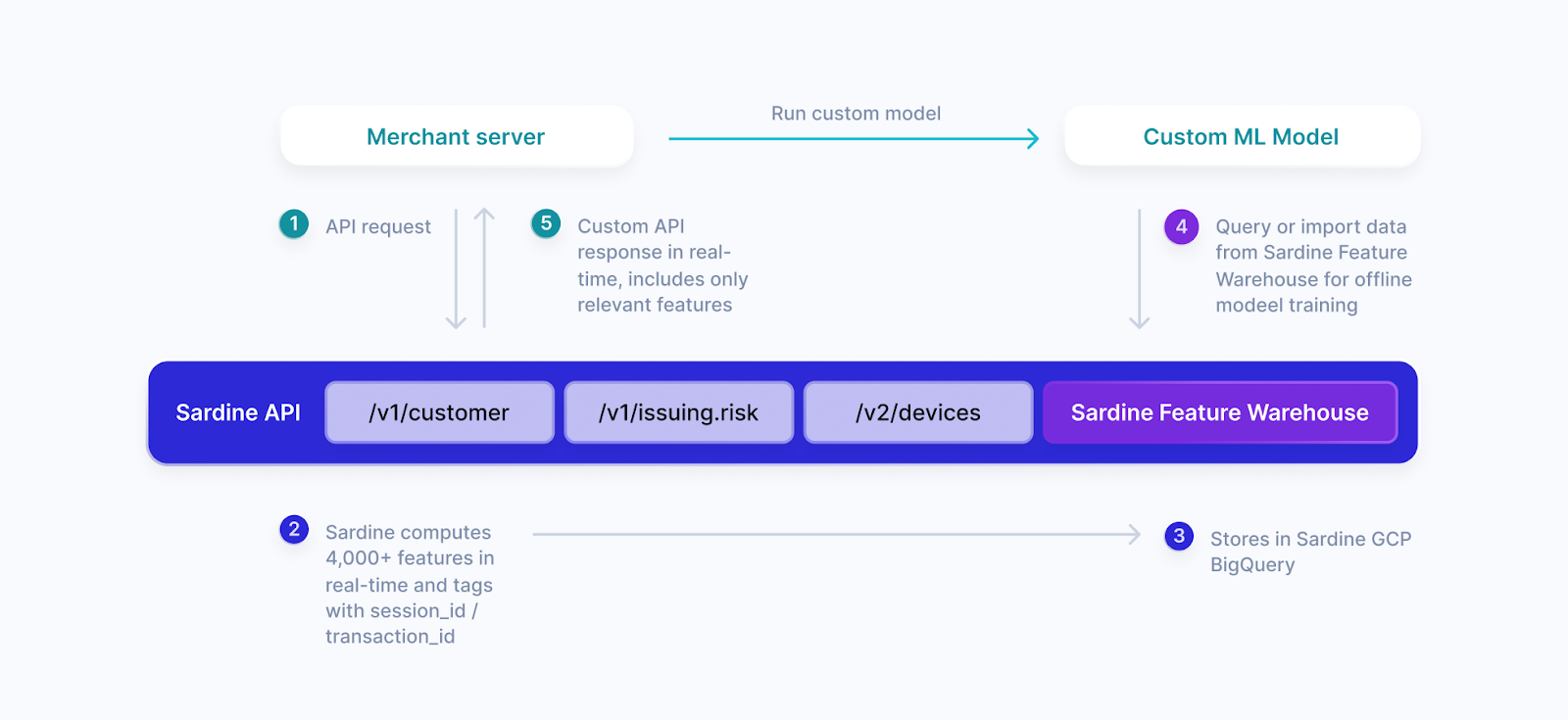
- When a Sardine client (merchant) requests any of the Sardine API endpoints
- Sardine computes over 4,000 machine-learned features in real-time and tags that to a user “session”
- The output of that session and the machine-learned features are fully stored in the data warehouse (BigQuery or Snowflake).
- Clients can then run custom ML models to query or import data from Sardine
It really is that simple.
But simple can be powerful.
When you consider the fraud and compliance squad almost never gets access to data and features when they want and how they want, this approach can be game-changing.
We expect to see a move towards feature stores across not just the risk spectrum but the entire Fintech infrastructure space.
By Data Scientists for Data Scientists
The Sardine feature store is by a data science team for data science teams. By centralizing, simplifying, and making accessible machine-learned features, we’re putting the power in the hands of those teams. No more black boxes. Just an extension of your team.
We’re here to help the fraud and compliance squad stop more fraud with more fine-grained features all from a single API and dashboard.
What will you do with the Sardine feature set?
We’re excited to see.
Contact us to learn more.