October 2023 Roundup - All risk problems are data problems
Hey Fraud & Compliance Squad 👋
The more time I spend at Sardine, the more I realize all risk problems are data science problems. So welcome to your everything is data edition of the Sardine newsletter. Think you’ll enjoy this one.
Btw: This month, we launched our GenAI assistant, Finley. Finley can answer questions like “Why did this rule fire?” or “Act as an analyst and please investigate user abcdefghi.”
But my favorite thing about Finley?
He’s named after a fluffy doggo and is 100% certified as a good dog.
- 📣 Rant: Old fashioned Machine Learning > GenAI for now
- 🛠 Tactical: AI upscaling for merchant data
- 🖼 Pattern: Remixing Data sources
Product: 🚢 The Sardine team keeps shipping. This month, we added 8 new features to our risk platform to help with rules, investigations, and case management. Read the blog for the full download.
Let’s dive in 🏊
ST.
📣 Monthly Rant: ML > GenAI for Fraud (for now)
You’re systematically not using ML enough. No matter how much you’re using it.
This Rant was triggered by a conversation I had with the CTO of a Neobank this week, who said there’s a giant opportunity to take a lead on machine learning for fraud detection as an industry.
Unsurprisingly, I agree.
I shared the Soups quote, “All risk problems are data science problems,” it resonated as someone who’s worked in big tech and operated with ML as a default and ML at a massive scale.
We don’t have that in financial services.
Yet.
Very few financial institutions, Neobanks, or e-commerce merchants have a tech platform that looks like Google or Meta. Those companies default to ML and neural networks to solve almost every problem. The best use of ML is in pockets and has relatively small models solving point issues. The larger financial institutions are building specialist teams to pull ML in-house and build that muscle.
But there’s a long way to go.
These models need to get much larger and more sophisticated to catch the rise in Fraud and Money Laundering facing the world. Having an ML model isn’t enough. Having the best-performing models consistently managed and updated by a world-class team is where we’re headed.
And because all risk problems are data science problems, we need to treat it that way.
All data science problems are data engineering problems.
This means we have to get the basics right.
We need a large, clean, and accessible data set.
That’s easy to say, hard to do.
Collecting a large data set in financial services means you’re collecting personal data and financial data and potentially sharing that. The compliance and governance overhead is not trivial.
There’s a ton of opportunity to get these basics right. Get a larger data set and leverage battle-tested ML techniques to deliver the ROI left on the table.
I’m not throwing shade at GenAI; it will inevitably be a game-changer (heck, we have Finley Live).
For fighting fraud, GenAI isn't the best tool. Machine learning is sufficient. And thats what we use at Sardine via both supervised machine learning models that extrapolate previous cases of fraud and identify other folks who are likely part of a fraud ring. We also use unsupervised models that are graph based that allow us to cluster together similar looking events or users into a potential ring.
However.
If we are talking about automating operations, whether fraud or compliance ops, then GenAI can be supremely helpful. Banks spend anywhere between 10-30% of their workforce on operations and with tools like AutoGPT, we can automate most of the mind numbing work they have to do day-in and day-out e.g. review cases automatically, create a SAR narrative automatically, or file a dispute to a chargeback automatically
And while that’s a huge cost saver, the big challenge with GenAI is it’s so early, the models, the value, and the use cases are still in flux.
As a data engineer, that’s hard to build (albeit rapidly changing!)
At Sardine we’re doing four things based on this hypothesis
- We give clients data warehouse and ML feature access. They can use our data and Sardine engineered features directly to train their models.
- We pull in data from 35+ data providers to enrich our data set. As data scientists, we’ve selected them for performance (coverage, quality, response SLA..)
- We’re building SardineX to create the most comprehensive and compliant (314(b), GLBA) data-sharing solution in the market. By data scientists for data scientists.
- We're building Finley as the ultimate Fraud operations co-pilot trained on our data, experience and platform.
➕ Fraud Squad: AI Upscaling for merchant data
Here’s a snippet from our breakdown of using Merchant data enrichment when battling fraud and chargebacks
For decades card issuers, banks, and Neobanks have been flying blind in their battle against fraud, resulting in lost revenue from false declines. The data they use is often confusing and means good customers get blocked, and fraudsters get away with their crimes. The fraud squad relies on ancient formats like ISO8583 messages and NAICS codes with card transaction data.
That changes today.
20/20 vision for Merchant POS Data
For card issuers like banks and Neobanks, merchant data was always unreliable and blurry.

ISO8583 message example
In this simplified transaction, a user has bought $15 worth of fast food at Yum. Yum! Brands is the parent company of KFC, Taco Bell, and Pizza Hut. So the first question is, which brand did they spend at?
Also, there are over 53,000 Yum Brands locations. Even Manhattan has 26 Taco Bells. How do we know if a user completed that purchase at that location? How do we know if it was a high fraud area? How do we know which brand it was?
If the fraud system relies on a Merchant Category Code, it could lead to either high fraud rates or false declines. Both are bad for business and bad for customers.
Here’s what it looks like with merchant intelligence combined with Sardine’s device data and brain.
- Collect the true location of the user's mobile phone at the time of purchase. That is something Sardine provides today via our Device Intelligence capabilities.
- Identify the true merchant identity and link it to the transaction. That is provided today via Spade’s merchant intelligence capabilities – with a single ID for every location or instance of a merchant, no matter how the name shows up in the descriptor or which Merchant ID is present
- Collect the true merchant location and link it to the transaction. That is provided today via with merchant intelligence capabilities.
- At the point of transaction authorization, combine these data points to provide a real-time fraud assessment. Sardine’s rule engine, dashboard, and 🧠 pull together any data source, enrich it, and make real-time decisions.
20/20 vision for e-Commerce
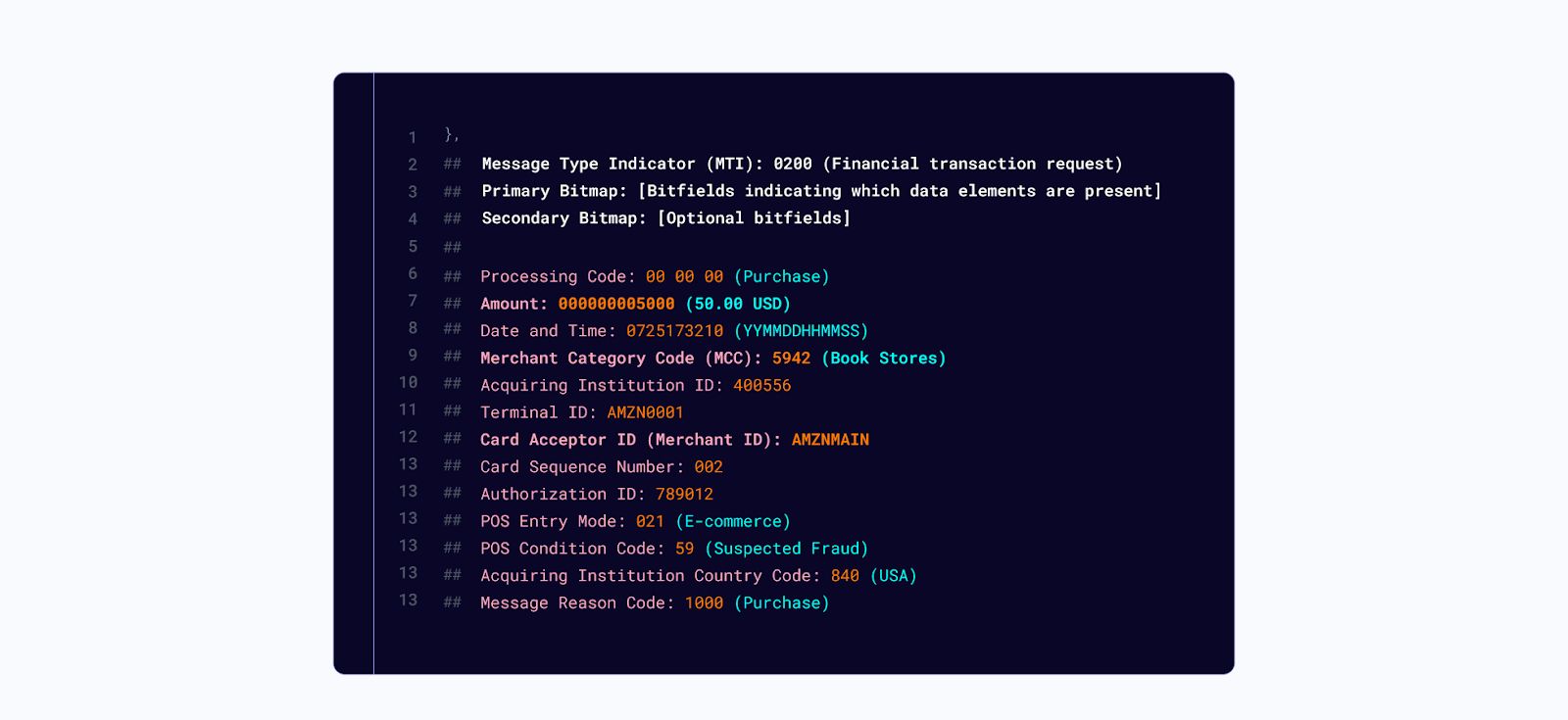
Here’s an e-commerce example
In this example, we see a user purchasing $50 on Amazon. But we don’t know if that's electronics, books, or digital content, each with its own fraud risk. The MCC Code is set to “book stores,” which doesn’t capture everything Amazon does. The transaction is suspected of fraud from the message, but how can we validate that? Might this lead to a false decline?
We can make this much higher resolution with Merchant data.
- Collect device history data. If this device has routinely behaved well with low fraud risk at multiple merchants, even if the card is new, we have higher confidence this is not a fraud.
- Collect user behavior signals. Is this new card purchase on this device typing, swiping, or tapping their keys in the same way they always do, or has something changed? If something has changed, this could be a fraud. If it’s the same, it likely isn’t.
- Sub-categorize the merchant. Was this an AWS transaction, an Amazon Prime subscription, or a Kindle purchase? The answer makes a meaningful difference to your decisioning.
- Build custom rules. Sardine allows users to create new rules using no-code or low-code quickly. Users can select from a vast array of features (which include data points submitted to the Sardine API, enrichments done by Sardine through consortium risk signals, and derived fields by Sardine’s proprietary models). Then, add outcome actions and submit the rule in Live or Shadow environments.
- Real-time risk scoring. At the point of transaction authorization, combine these data points to provide a real-time fraud assessment. Then, approve, deny, or flag a transaction for review.
Get a real-time view of the true merchant ID, pull in any data you like, build a custom rule, and decide whether to approve the transaction auth.
Less false positives. More conversion. Less fraud.
Lovely.
If you want to read the full piece you can check it out here
And if you AI upscaling for merchant risk, get in touch. Either hit reply or email us hello@sardine.ai
🕵️ Pattern: Why we need to remix data sources
“Data enrichment” sounds so dull but is incredibly powerful.
The Power of Data Enrichment:
The adage "quality over quantity" has never been truer in a world drowning in data. Enter data enrichment – enhancing raw data with additional information to provide a clearer picture. It's like having a magnifying glass that highlights the most crucial details.
All risk problems are data science problems.
But it's not just about magnifying; it's about highlighting the right details.
You need the best data from the best providers to be available when you need it in the form you need it. This could be phone, email, address, credit card, bank account, and social security numbers (SSN) or local central IDs.
We always advise, “Don’t just aggregate data; curate it.” For example, we picked 35 specialized providers in our arsenal – all vetted by our in-house fraud and compliance squad – we’re on a mission to provide data that matters.
Whether it's data from open banking or those elusive signals from a "bank consortia," we’re working hard to get it because it gets results.
One example: The Gold in Bank Consortia Data
In my first week at Sardine, our co-founder Adi told me, “If you could have told me in my Fintech days we could have Bank Consortia data, I’d have been blown away.”
So, why all the fuss about bank consortia data? Imagine spotting a potential fraudster not just by their current actions but by delving into a history where they might have been implicated before.
This data acts like a memory, recalling instances that help us make better decisions now. Combine this with open banking data, email history, or telco data, and you can stop a bad actor at onboarding before they become a problem or decline the right transaction at checkout.
How we select data providers.
We need three things to make the data useful for the fraud squad.
- Performance: Data and the providers must deliver. The primary KPIs are better check-out conversion, reducing chargebacks, and manual work. Data providers need to deliver, and that’s our key metric when selecting providers.
- Curation: Not All Data is Created Equal. In the information age, discernment is key. At Sardine, each data point and provider is collected and vetted. Our experienced team ensures that the data you rely on is relevant and accurate.
- Customization: We get it; every business is unique. Hence, the choice is yours whether you want to consume our enriched data via an API or craft custom rules through our intuitive dashboard. We provide the tools; you wield them.
Combining signals is the key
Imagine a fraudster has stolen a phone. They’d pass the phone number check and possibly name and address if those data points are stolen. But what about the email? What about the device they’re using? You could miss the crucial data point without pulling it all together.
The nature of detection is looking for needles in the haystack. This happens to be something AI is uniquely good at. It spots inconsistencies, but like all data science, it requires lots of data and high-quality data.
Data science needs data access
To find needles in a haystack, data scientists need data warehouse access, but that’s surprisingly hard to get. Most fraud and risk platforms provide a risk score but might not share the data. The problem is every business has a unique risk profile. Their data scientists are often best placed to build new models, but it's still rare to get access to the right data with the right quality.
Putting it all together
Curate the right data providers, benchmark them for performance, and make sure data scientists have the access they need to solve the problems the business is facing.
If you enjoyed this you’ll find the full piece here
That’s all, folks 👋
Love this stuff? You can say thanks by getting yourself a demo of Sardine.
Still here? Why not say hello on Twitter or LinkedIn?
🧙Quest: Send us your hardest fraud problem to solve. What are you stuck on? Sardines swim together.
We’re here to help.